Machine Learning Pipelining: Unlocking Efficient AI for Businesses
Understanding ML Pipelining
- Machine learning (ML) pipelining is a critical technology that helps organizations streamline AI workflows.
- It enables efficient development and deployment of ML models, making processes scalable.
Importance of ML Pipelining
- ML Pipelining automates the end-to-end process of developing and deploying machine learning models.
- This automation covers various stages: Data collection, Data preprocessing, Model training, Model evaluation, Model deployment.
ML Pipelining for businesses
- ML Pipelining is simplifies and automates complex tasks involved in ML model development and deployment, ultimately enhancing efficiency and scalability.
Experience the Future with Us
By organizing all steps into a coherent pipeline, companies can ensure that their ML workflows are reproducible, scalable, and easier to manage.
The Benefits of ML Pipelining
Efficiency and Speed
Automating repetitive tasks within the ML workflow significantly reduces the time required to develop and deploy models. This allows businesses to respond more rapidly to market changes and customer needs.
Scalability
Pipelining facilitates the handling of large volumes of data and complex modelling processes, enabling businesses to scale their AI solutions as they grow without compromising on performance.
Consistency and Quality
By standardizing the ML workflow, pipelining ensures that every step of the process meets the business’s quality standards, leading to more reliable and accurate models.
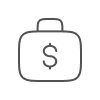
Cost Reduction
Through automation and efficiency, ML pipelining can significantly reduce the costs associated with manual data handling and iterative model tuning.
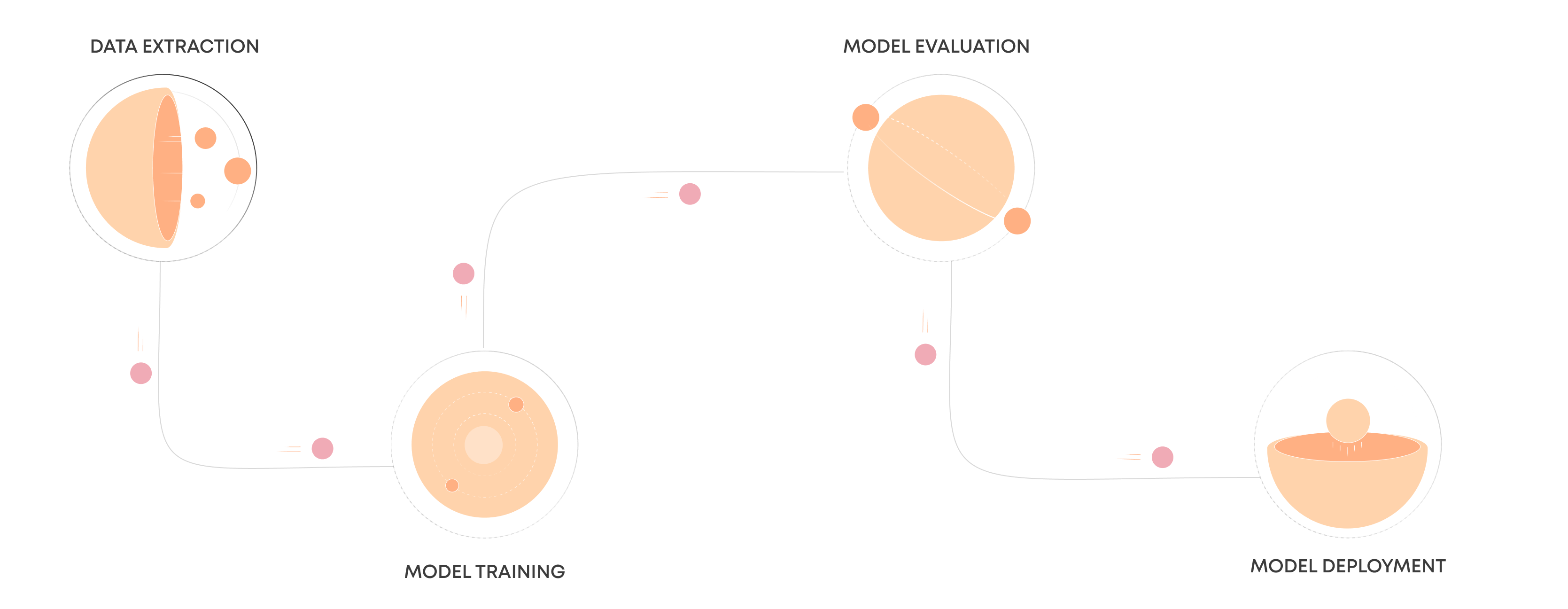
ML Pipeline
01/

Data collection & pre-processing
The journey begins with data collection and pre-processing, a fundamental step in which raw data is collected from various sources such as databases, online repositories or sensors. This raw data often contains inaccuracies, missing values or irrelevant information that requires thorough cleansing and normalization to ensure it is in a usable format. Pre-processing transforms this raw data into a structured form and makes it suitable for analysis by addressing issues such as missing data, normalization of data scales and coding of categorical variables, providing a solid foundation for accurate model training.
02/

Feature Enginneering
The journey begins with data collection and pre-processing, a fundamental step in which raw data is collected from various sources such as databases, online repositories or sensors. This raw data often contains inaccuracies, missing values or irrelevant information that requires thorough cleansing and normalization to ensure it is in a usable format. Pre-processing transforms this raw data into a structured form and makes it suitable for analysis by addressing issues such as missing data, normalization of data scales and coding of categorical variables, providing a solid foundation for accurate model training.
Following preprocessing, feature engineering takes the stage, acting as a bridge between raw data and predictive models. This process involves extracting, selecting, and transforming data attributes (features) to enhance the model’s predictive power. Effective feature engineering might include creating new features that capture underlying patterns in the data, selecting the most relevant features to reduce complexity, or transforming features to better align with model assumptions. This step is crucial for boosting model performance, as the right features can significantly improve how well a model can learn from the data.
03/

Model Training
With the data prepared and the best features at hand, we move to model training, where algorithms learn from the data. This phase involves feeding the processed data into various machine learning algorithms to build models that can predict outcomes or classify data into different categories. The choice of algorithm depends on the task at hand (e.g., regression, classification) and the nature of the data. During training, the model iteratively adjusts its parameters to minimize errors in predictions, effectively learning the patterns within the training dataset.
04/

Evaluation & Validation
Once a model is trained, evaluation and validation are critical for assessing its performance. This involves using metrics like accuracy, precision, recall, or mean squared error to measure how well the model predicts on data it hasn’t seen before. Validation techniques such as cross-validation help ensure that the model’s performance is consistent across different data subsets, guarding against overfitting where a model learns the training data too well but performs poorly on new data. This step confirms whether the model is ready for real-world deployment or requires further tuning.
05/

Deployment Stage
Successful models then move into the deployment phase, where they are integrated into operational systems to provide initial insights or predictions in a real-world environment. Deployment can vary widely, from embedding the models into existing software applications to deploying them on cloud-based platforms for scalability. At this stage, the model interacts with new data in real-time or batch processes and provides valuable insights or automated decisions to improve business processes or user experiences.
06/

Monitoring & Maintenance
Finally, monitoring and maintenance ensure the longevity and relevance of deployed models. Over time, models can drift from their initial performance due to changes in underlying data patterns or external conditions. Regular monitoring allows for the detection of such changes, prompting model retraining or adjustment as necessary. Maintenance also involves updating the data preprocessing steps or features to keep the model accurate and relevant, ensuring it continues to meet its objectives in a changing environment.
Leveraging ML Pipelining for Competitive Advantage
By adopting ML Pipelining, companies can not only optimise their AI workflows but also foster innovation and agility within their operations. This technology allows businesses to deploy sophisticated ML models that can predict customer behaviour, optimise operational processes, and provide actionable insights, thereby driving growth and efficiency.