The future of Company Information: Knowledge Base Search
An open source document from Volkswagen Group was taken as an example of how the Retrieval Augmented Generation (RAG) Knowledge Base Search works. This document is publicly available for viewing and download. Note: we are not affiliated with this organization (it’s just a sample pdf we can find online…)
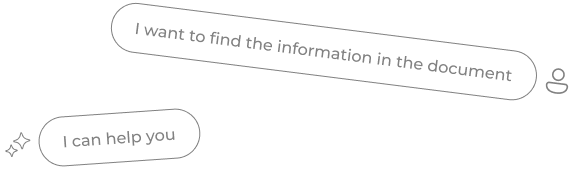
Try asking a question for the knowledge base search bar
How it works
01/
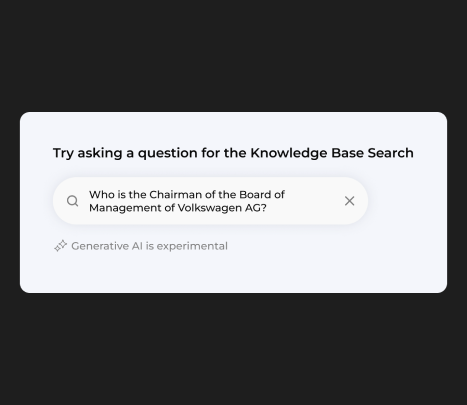
The RAG stores your company documents securely in a vector store
02/
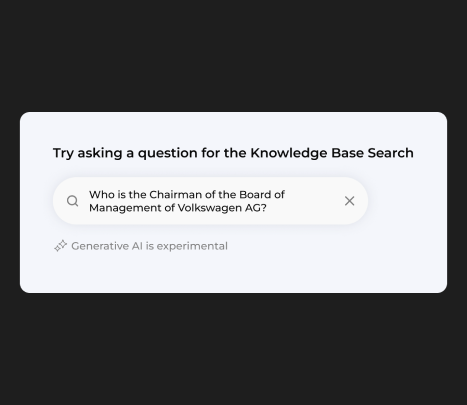
Users write the question of interest from this document.
03/
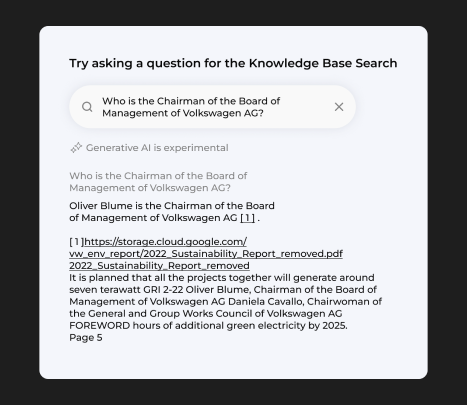
Search Bar gives a clear answer and can show which page has detailed information on the question asked without hallucinations.
Visualizing Our Approach
At the heart of our innovative platform is the Retrieval Augmented Generation (RAG) system. Imagine a digital librarian that can understand and search through not just books but also a universe of digital content, including images and text. That’s what our RAG system does — it carefully picks out the most relevant pieces of information from a vast array of data. Just like how a chef chops up ingredients to create a gourmet dish, our system breaks down information into bite-sized pieces. These pieces, or “chunks,” are then transformed into a special code, known as embeddings, that our system can quickly understand and analyze. Think of these embeddings as entries in a super-fast digital library card catalog, all stored in a place we call the vector database. When you ask a question, our system turns your words into a similar code and quickly finds the best match in this catalog. It’s like finding the perfect book in a library, but at lightning speed.
The magic happens when our Language Learning Model (LLM) comes into play. It’s like a wise sage that takes the information and crafts a response that’s not just correct but also tailored to the context of your question. This ensures you get answers that make sense and provide real insight. By combining the careful prep work of gathering and organizing data with the creative touch of our LLM, we give you answers that are more than just data points — they’re meaningful, relevant, and crafted with care. It’s this combination of data precision and human-like understanding that takes your customer experience to the next level, making every interaction with our service feel intuitive, helpful, and impressively smart.
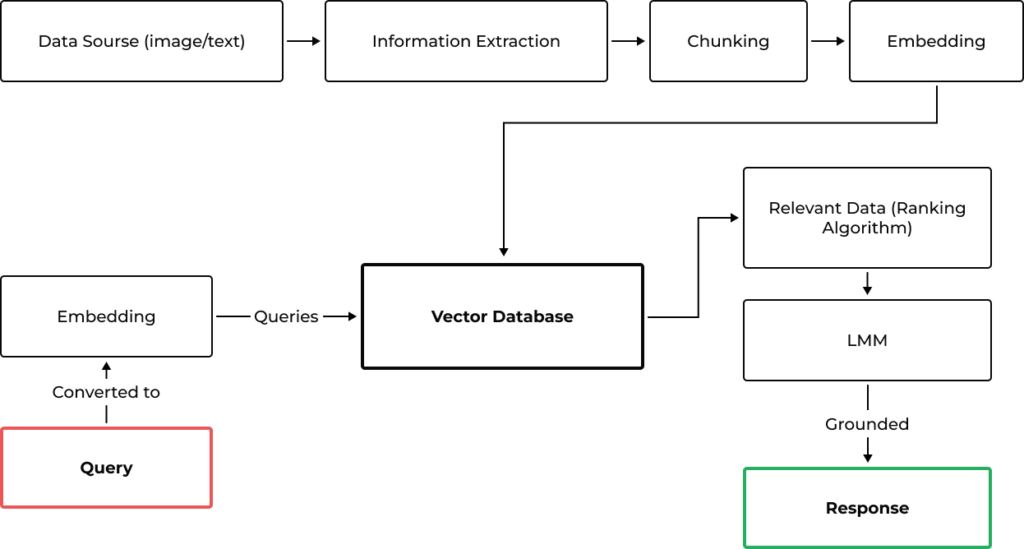
An Example of Vertex AI search and Conversation
Data Extraction and Embeddings
The data is first extracted, chunked (make small corpus of text), and then embeddings (i.e these chunks of text are assigned numerical value also called vectors) are created.

Query Responses
The query will return responses by using a relativity algorithm to find the embeddings closer to the embeddings of question and reply with suitable answer.
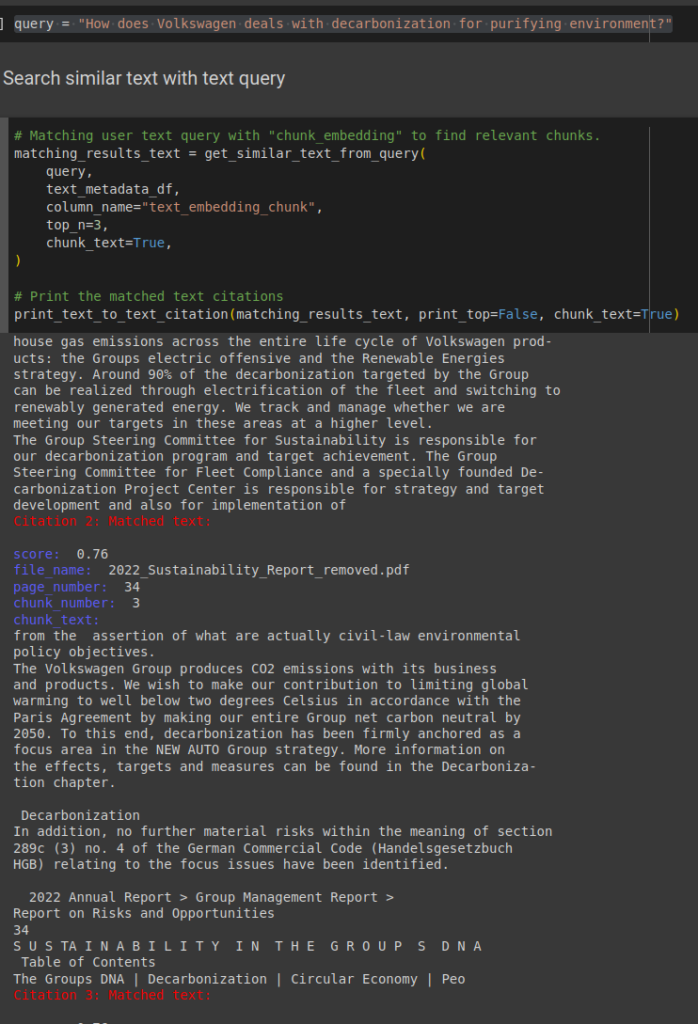
Image Query Responses
If you like to ask a query based on image, e.g you might have an image of table, you simply insert the image and the vector database will find the relevant image from the documents and return it to you. Here we will use ‘Multimodal Learning ‘
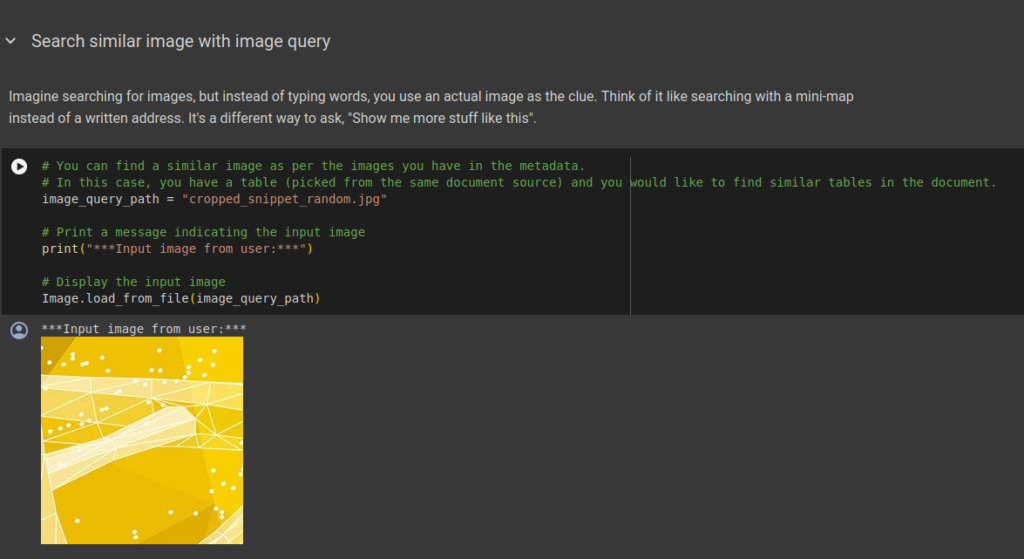
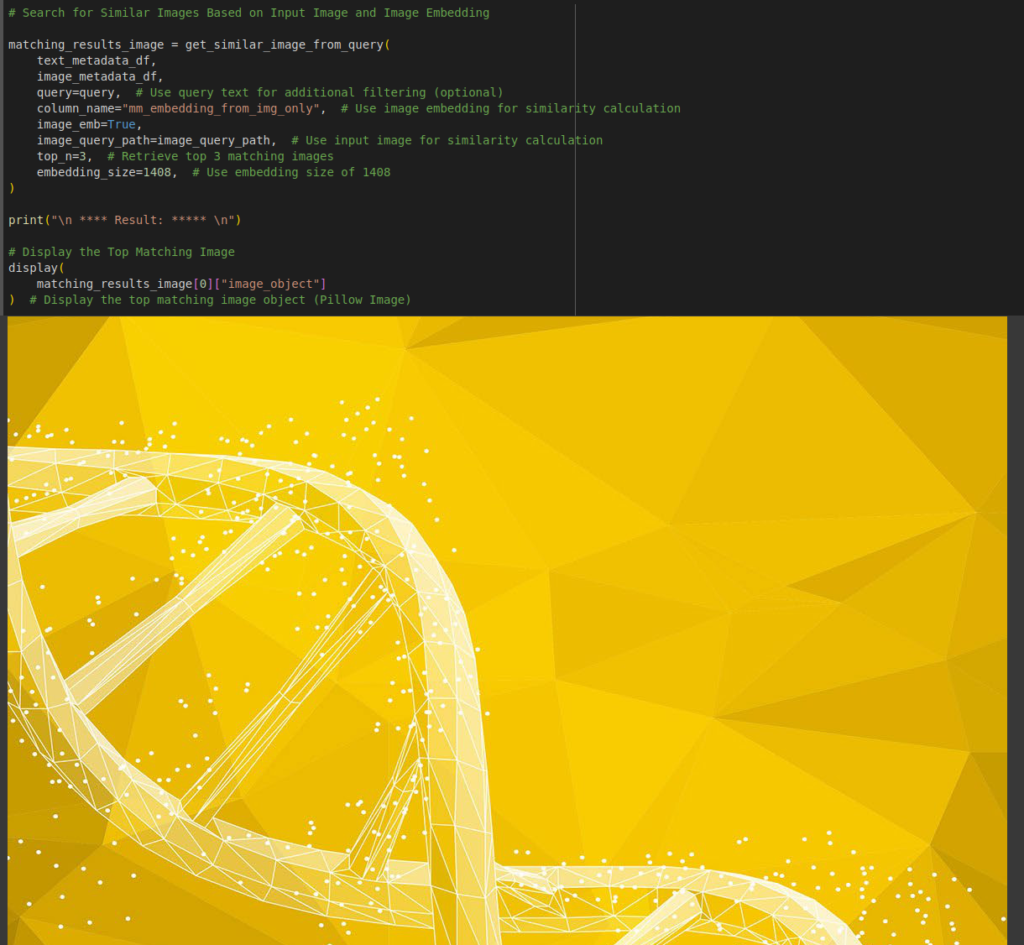
Grounded Responses
RAG lets us ground the user responses to actual matched text without any hallucinations. If the relevant information is not present, The app will not provide any random information, rather it will respond with an apologetic message that the required information is not provided. As seen in the reference pdf file; there is no mention of Mercedes in the document. This is particularly useful when consisted company related information is required.
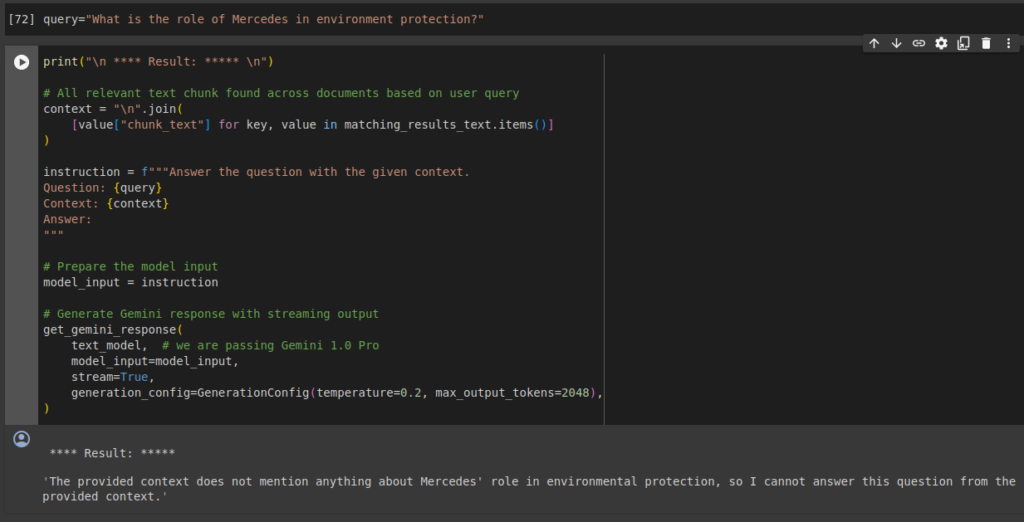